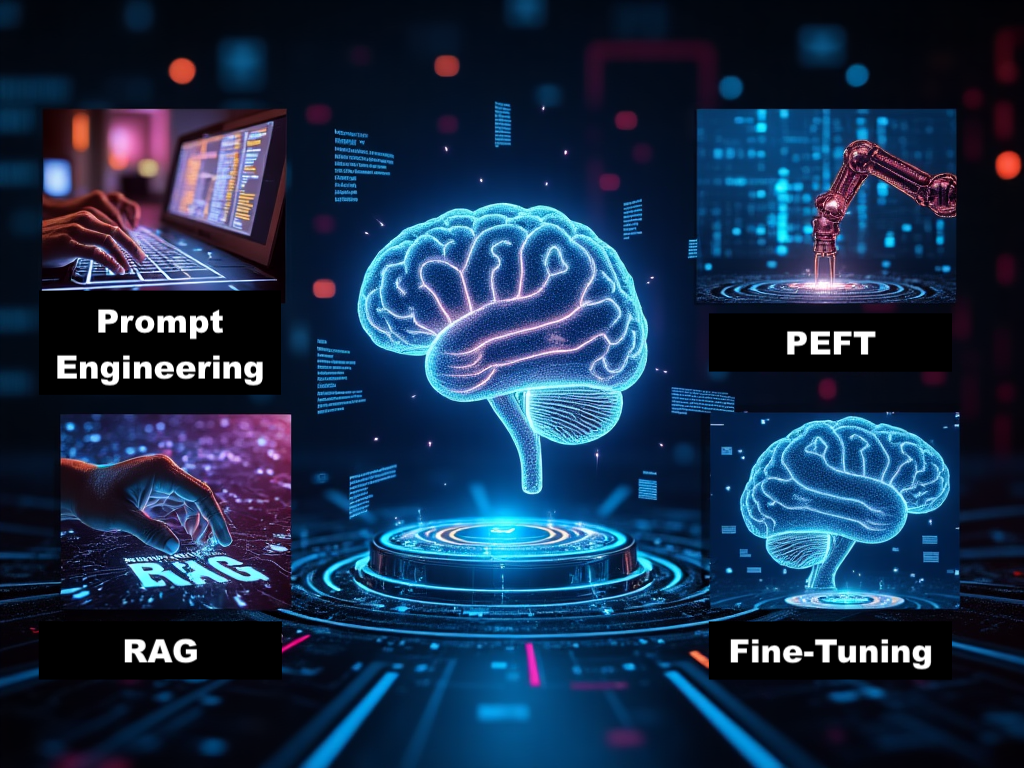
A large language model or LLM is a type of machine learning model designed for natural language processing or NLP. These models have an extremely high number of parameters (trillions as of this writing) and are trained on vast amounts of human-generated and human-consumed data. Due to their extensive training on this data, LLMs develop predictive capabilities in syntax, semantics, and knowledge within human language. This enables them to generate coherent and contextually relevant responses, giving the impression of intelligence.
Read More