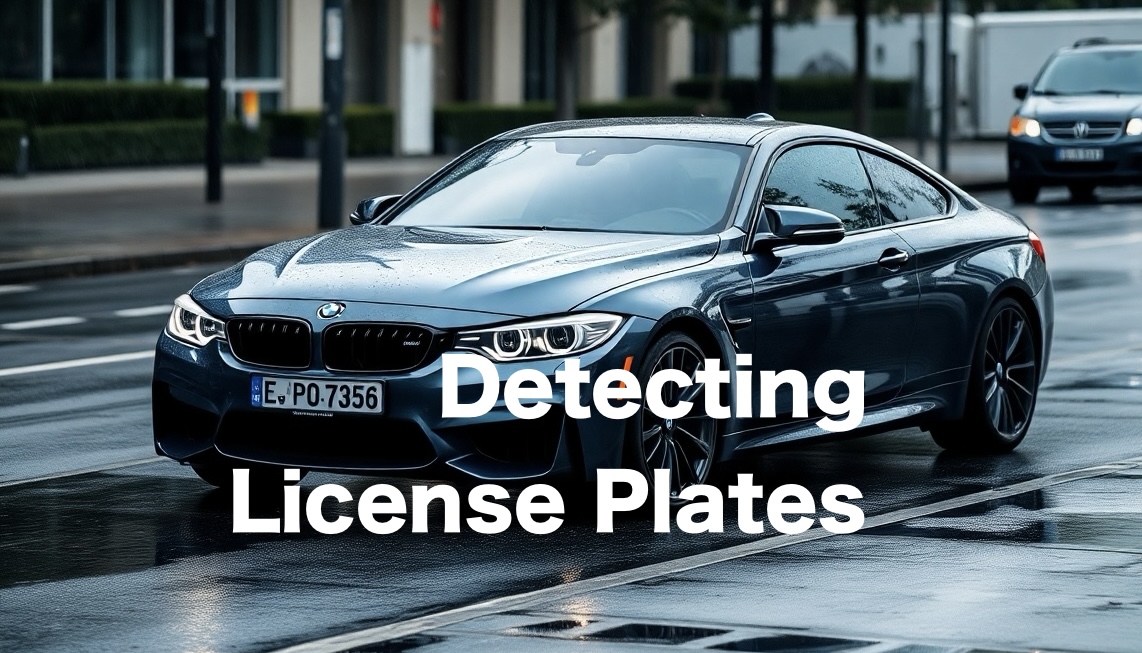
The nice thing about ChatGPT and similar systems is that the complexity of AI/ML functionality is hidden behind a friendly natural language interface. This makes it easily reachable to the masses. But behind this easy to use facade is a lot of advanced functionality that involve a sequence of data processing steps called a pipeline. An AI-powered business card reader, for example, would first detect text and then recognize the individual letters within the context of the words they belong to. A license plate reader would be similar. Detection is an important process that you often need in your AI/ML projects. And that’s why we will be looking at YOLO.
Read More